Ensuring Safety in the Age of Artificial Intelligence: A Comprehensive Review of AI Safety Applications
8 min readArtificial intelligence (AI) has emerged as a transformative technology with the potential to revolutionize various industries and domains. From healthcare and finance to transportation and cybersecurity, AI is making significant advancements, offering unprecedented opportunities for innovation and efficiency. However, as AI continues to advance, it is crucial to address the potential risks and ensure its safe and responsible deployment. This blog aims to provide a comprehensive review of AI safety applications, highlighting the measures and techniques employed to mitigate risks and ensure the safe use of AI systems.
Also Read: E-Books: Introduction to Environmental Management


Understanding AI Safety
Before delving into the details of AI safety applications, it is important to establish a common understanding of what AI safety entails. AI safety refers to the measures and practices implemented to minimize the risks associated with AI systems. It encompasses various aspects, including transparency, robustness, human-AI interaction, ethical considerations, regulation, and collaborative approaches.
Ensuring Transparency and Explainability
Transparency and explainability are fundamental to building trust and understanding AI systems. Interpretable machine learning techniques aim to make AI models more understandable and interpretable by humans. Explainable AI techniques, such as generating explanations for AI decisions, help users understand the rationale behind AI outputs. Model documentation and reporting provide insights into the development process, data sources, and performance metrics of AI systems. Furthermore, accountability and regulatory compliance ensure that AI systems adhere to ethical and legal standards.
Robustness and Security
AI systems must be robust and secure to withstand potential attacks and ensure reliable performance. Adversarial attacks and defences focus on identifying vulnerabilities and developing countermeasures against malicious manipulations of AI systems. Secure and privacy-preserving AI techniques safeguard sensitive data and protect user privacy. Data integrity and bias mitigation aim to address biases in training data and ensure fair and unbiased AI outcomes. Robustness testing and verification processes evaluate the performance and reliability of AI systems under various scenarios.-AI Interaction and Ethical Considerations
As AI systems become more integrated into our daily lives, ensuring smooth human-AI interaction and addressing ethical considerations become crucial. User-centric design and usability prioritize the development of AI systems that are intuitive and user-friendly. Human-AI collaboration and teamwork explore ways to leverage the strengths of both humans and AI systems for improved outcomes. Fairness, bias, and discrimination must be actively addressed to prevent AI systems from perpetuating societal inequalities. Ethical frameworks for AI safety provide guidelines and principles to ensure responsible and ethical AI development and deployment.
AI Safety in Autonomous Systems
Autonomous systems, such as self-driving cars, robots, and unmanned aerial systems (UAS), pose unique safety challenges. Safety engineering for autonomous vehicles focuses on developing robust and fail-safe systems to prevent accidents and ensure passenger safety. Robotics and industrial automation require stringent safety measures to protect human workers and ensure safe operations. UAS and drones require strict regulations and safety protocols to prevent accidents and protect public safety.
Regulation and Governance
Effective regulation and governance play a vital role in ensuring AI safety. Legal and policy considerations must keep pace with technological advancements to address potential risks and ensure responsible AI use. International standards and guidelines provide a framework for organizations and governments to develop and implement AI safety measures. Government initiatives and regulations establish safety requirements and promote responsible AI practices. Industry self-regulation and best practices encourage organizations to prioritize safety in AI development and deployment.
Also Read: What Is The Difference Between Incidents and Accidents?
Collaborative Approaches to AI Safety
Addressing AI safety requires collective efforts from researchers, policymakers, industry leaders, and the public. Research and academic collaborations foster innovation and knowledge sharing in AI safety. Public-private partnerships encourage collaboration between government entities and industry players to develop and implement safety standards. Open-source and community involvement promote transparency, accountability, and the sharing of best practices. Data-sharing initiatives facilitate the development of robust AI systems by providing access to diverse and representative datasets.
Also Read: Managing Risk in Healthcare
Case Studies and Real-World Implementations
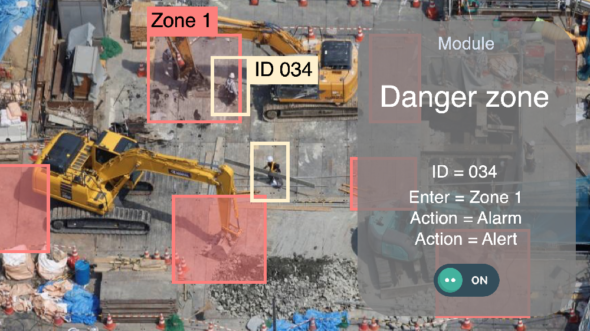
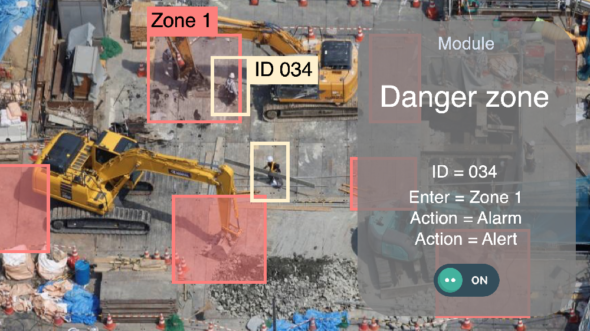
Real-world examples demonstrate the practical application of AI safety measures. In healthcare, AI safety focuses on ensuring the accuracy and privacy of medical data and the reliability of AI-assisted diagnoses and treatments. AI for financial risk management involves developing robust algorithms to detect fraudulent activities and mitigate financial risks. AI in critical infrastructure protection addresses the security and safety concerns associated with AI-driven systems in essential infrastructure sectors. AI for cybersecurity focuses on developing advanced threat detection and prevention mechanisms.
Here are additional examples of real-world implementations of AI safety measures across various domains:
- AI Safety in Autonomous Vehicles:
- Collision Avoidance Systems: AI algorithms analyze sensor data in real time to detect potential collisions and trigger prompt actions, such as automatic braking or steering adjustments.
- Fail-Safe Mechanisms: Advanced safety measures ensure that autonomous vehicles can handle unexpected situations, such as system failures or adverse weather conditions, by safely transitioning control back to human drivers or initiating emergency protocols.
- AI Safety in Healthcare:
- Patient Data Privacy: AI systems used in healthcare are designed with robust privacy measures to protect sensitive patient data, ensuring compliance with regulations like HIPAA (Health Insurance Portability and Accountability Act).
- Bias Detection and Mitigation: AI algorithms are employed to identify and address biases in medical data and decision-making processes, ensuring equitable treatment for patients from diverse backgrounds.
- AI Safety in Finance:
- Fraud Detection: AI-powered algorithms analyze vast amounts of financial data to identify patterns indicative of fraudulent activities, enabling timely detection and prevention of financial fraud.
- Risk Assessment and Compliance: AI models assist financial institutions in assessing and managing risk by analyzing market trends, regulatory changes, and transaction data, helping ensure compliance with regulations.
- AI Safety in Cybersecurity:
- Threat Detection and Response: AI algorithms analyze network traffic, system logs, and user behaviour patterns to detect and respond to cybersecurity threats, enabling proactive defence against cyberattacks.
- Vulnerability Assessment: AI-powered tools automatically scan software code and IT infrastructure to identify potential vulnerabilities, helping organizations address security weaknesses before they can be exploited.
- AI Safety in Aviation:
- Predictive Maintenance: AI algorithms analyze sensor data from aircraft systems to predict maintenance needs, identifying potential failures in advance and reducing the risk of in-flight malfunctions.
- Air Traffic Management: AI-based systems optimize air traffic flow, reducing congestion and the potential for collisions, ensuring the safe and efficient movement of aircraft.
- AI Safety in Manufacturing:


- Quality Control: AI-powered visual inspection systems detect defects and anomalies on production lines, ensuring that only high-quality products reach the market.
- Worker Safety: AI technologies, such as computer vision and wearable devices, monitor worker activities and environments, alerting them to potential safety hazards and preventing accidents.
These examples demonstrate the diverse applications of AI safety measures across different sectors, highlighting the importance of implementing robust safeguards to ensure the safe and responsible use of AI technologies.
Here are additional examples of AI safety measures in various industries:
- AI Safety in Energy:
- Predictive Maintenance for Power Grids: AI algorithms analyze data from power grid sensors to identify potential failures or anomalies, allowing for proactive maintenance and preventing power outages.
- Safety Monitoring in Oil and Gas: AI systems monitor operations in hazardous environments, detecting safety risks and providing real-time alerts to prevent accidents or equipment failures.
- AI Safety in Retail:
- Customer Privacy and Data Protection: AI systems used in personalized marketing or recommendation engines are designed to protect customer privacy and ensure compliance with data protection regulations.
- Product Safety and Quality Assurance: AI-powered visual inspection systems verify product quality, detect defects, and ensure compliance with safety standards before items are made available for sale.
- AI Safety in Agriculture:
- Crop Monitoring and Disease Detection: AI algorithms analyze drone or satellite imagery to monitor crop health, identify diseases or infestations, and enable targeted interventions for healthier yields.
- Autonomous Agricultural Machinery: Safety measures are implemented in AI-driven autonomous machinery, such as tractors or harvesters, to prevent accidents and ensure the well-being of farmers and workers.
- AI Safety in Education:
- Adaptive Learning Systems: AI-powered adaptive learning platforms ensure the safety and well-being of students by monitoring their progress, providing personalized feedback, and identifying potential learning difficulties or emotional distress.
- Content Filtering and Child Protection: AI algorithms can analyze and filter content to protect students from inappropriate or harmful material, ensuring a safe online learning environment.
- AI Safety in Law Enforcement:
- Facial Recognition Ethics and Bias Mitigation: AI systems used in facial recognition are designed with ethical considerations, such as bias detection and mitigation, to avoid discriminatory outcomes and protect privacy.
- Predictive Policing Accuracy and Accountability: AI algorithms used in predictive policing are subject to rigorous testing and evaluation to minimize biases, ensuring accurate and accountable crime prediction and prevention.
- AI Safety in Space Exploration:
- Autonomy and Redundancy in Spacecraft: AI systems in space missions incorporate safety measures such as redundancy and fail-safe mechanisms to ensure the reliability and safety of spacecraft operations.
- Collision Avoidance in Satellite Networks: AI algorithms analyze orbital data and predict potential collisions, enabling timely adjustments of satellite trajectories to prevent accidents and space debris formation.
These examples illustrate how AI safety measures are being implemented across a wide range of industries, emphasizing the importance of considering safety aspects when integrating AI technologies into various applications. By incorporating robust safety measures, these industries can harness the benefits of AI while prioritizing the well-being of individuals and the environment.
Improve Pipeline Safety Management with Aerial Data
Conclusion
As AI continues to evolve and permeate various aspects of our lives, ensuring its safety becomes paramount. This blog has provided an in-depth exploration of AI safety applications, covering transparency, robustness, human-AI interaction, autonomous systems, regulation, collaborative approaches, and real-world implementations. By adopting these measures and considering ethical considerations, we can harness the potential of AI while minimizing risks and ensuring the well-being of individuals and society as a whole. As this field progresses, ongoing research, collaboration, and responsible governance will play a pivotal role in shaping the future of AI safety. With concerted efforts from researchers, policymakers, industry leaders, and the public, we can navigate the complex landscape of AI and build a safer and more trustworthy AI-powered world. By prioritizing AI safety, we can unlock the full potential of AI while safeguarding against potential risks and ensuring the benefits are enjoyed by all. Let us embrace this transformative technology responsibly and work together to shape a future where AI enhances our lives while prioritizing safety and well-being.
Also Read: Keys to Sustainable Safety and Ergonomics
For more safety Resources Please Visit Safetybagresources